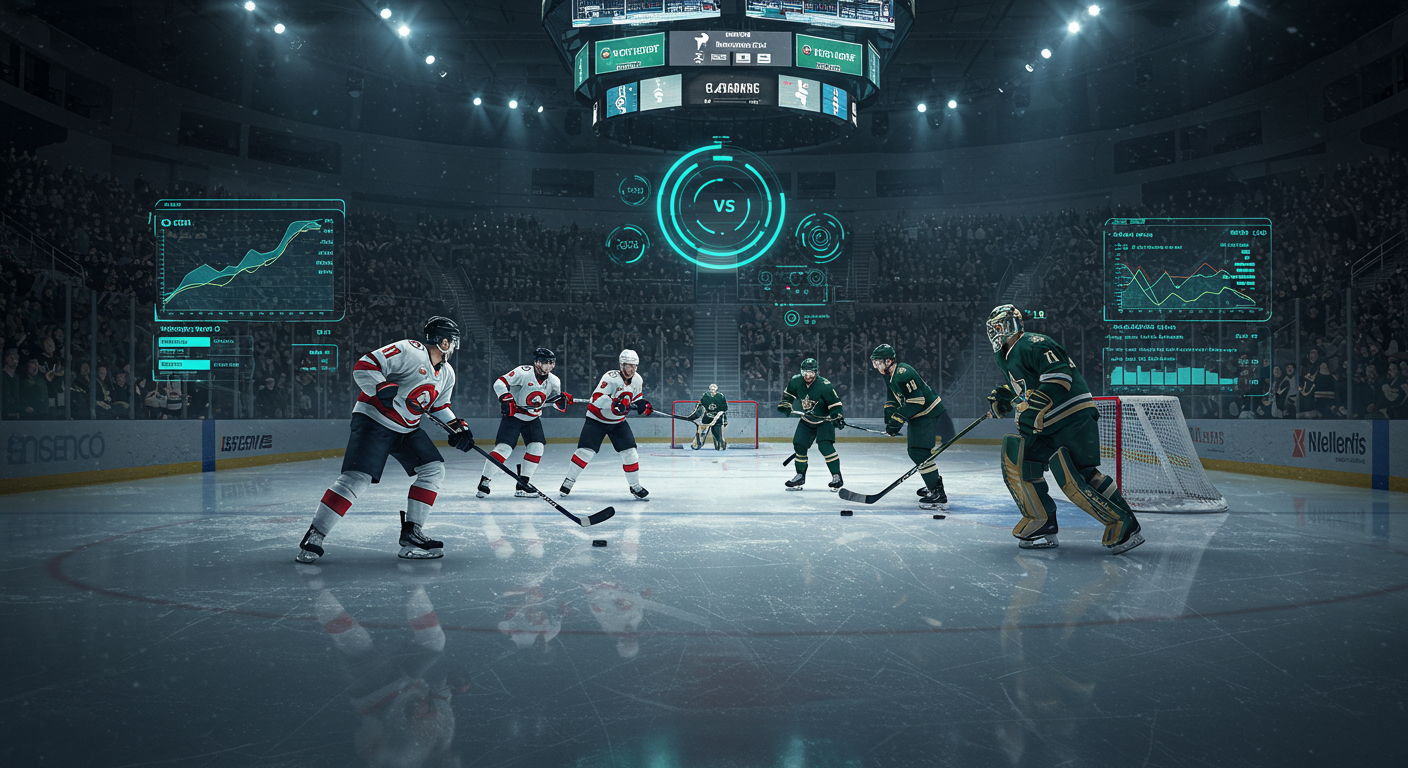
Introduction
In the fast-paced realm of sports simulations—particularly in hockey—digital twins offer the ability to simulate nearly every aspect of the game. By creating virtual replicas of players, strategies, and even the ice rink itself, coaches and players can analyze, predict, and optimize performance on a game-by-game basis with remarkable accuracy.
Broadly, there are four types of analytics used in hockey: descriptive, diagnostic, predictive, and prescriptive.
-
Descriptive analytics examine historical player and performance data to identify past patterns and trends.
-
Diagnostic analytics delve into the “why” behind these patterns, pinpointing root causes.
-
Predictive analytics leverage historical data, statistics, and machine learning to forecast future events or outcomes.
-
Prescriptive analytics build on all three, using optimization techniques to recommend specific actions or strategies.
A digital twin takes prescriptive analytics a step further by creating a virtual “doppelganger” of a player or team based on real-time data, enabling coaches and players to evaluate how they might function or perform in a digital environment before making real-world decisions.
Using a Digital Twin in Hockey
In hockey, a digital twin can be used to create digital representations of players, arenas, and strategies. These representations help coaches and athletes predict behaviors, evaluate performance, and make informed decisions that optimize game outcomes. For instance, coaches can model different plays, line combinations, and tactical approaches in a virtual scenario before deploying them on the ice. Outcomes vary depending on each opponent’s style, performance trends, and even whether the game is at home or away.
A digital twin also allows for advanced planning: predicting how specific players will behave in certain situations, how opponents might react, and which tactical adjustments could be most effective. Functioning as a “simulation arena,” the digital twin provides real-time insights that can guide decisions made during an actual game.
Below are three scenarios where digital twins can enhance strategic decision-making with a data-driven approach.
1. Play Digital Twin (e.g., faceoff plays)
A “play twin” is the most fundamental application of digital twin technology, focusing on a single play type within a game context. It evaluates how well a specific play—like a faceoff formation, breakout pattern, or offensive zone entry—performs against a particular opponent. By simulating these plays ahead of time, coaches gain insight into effectiveness and potential improvements, ultimately refining practice plans and play selections based on digital simulation outcomes.
2. System Digital Twin (e.g., forecheck systems)
System twins model how different players and line combinations perform as a cohesive unit. This broader view allows coaches to test various systems (e.g., a 1-2-2 forecheck, power play, penalty kill) and determine which configurations yield the best results against specific opponents. Through these simulations, teams gather valuable data to refine large-scale strategies, discover new systems, and optimize existing ones.
3. Process Digital Twin (complete game strategy)
At the highest level, process twins integrate multiple system twins into one holistic strategy. By connecting all plays and systems into a unified simulation, coaches can develop a complete game plan before stepping onto the ice. This top-level approach offers the most comprehensive perspective on team dynamics, facilitating informed, data-driven decisions in real time.
Conclusion
Digital twins have the potential to transform hockey by providing deeper insights into player performance and enabling teams to fine-tune tactics and strategies with a high degree of precision. By translating extensive performance data into accurate simulations, digital twins represent a new era of data-driven decision-making for both player development and coaching. As the technology matures, its impact on the sport will only grow, ensuring that teams and players reach new levels of competitive advantage.