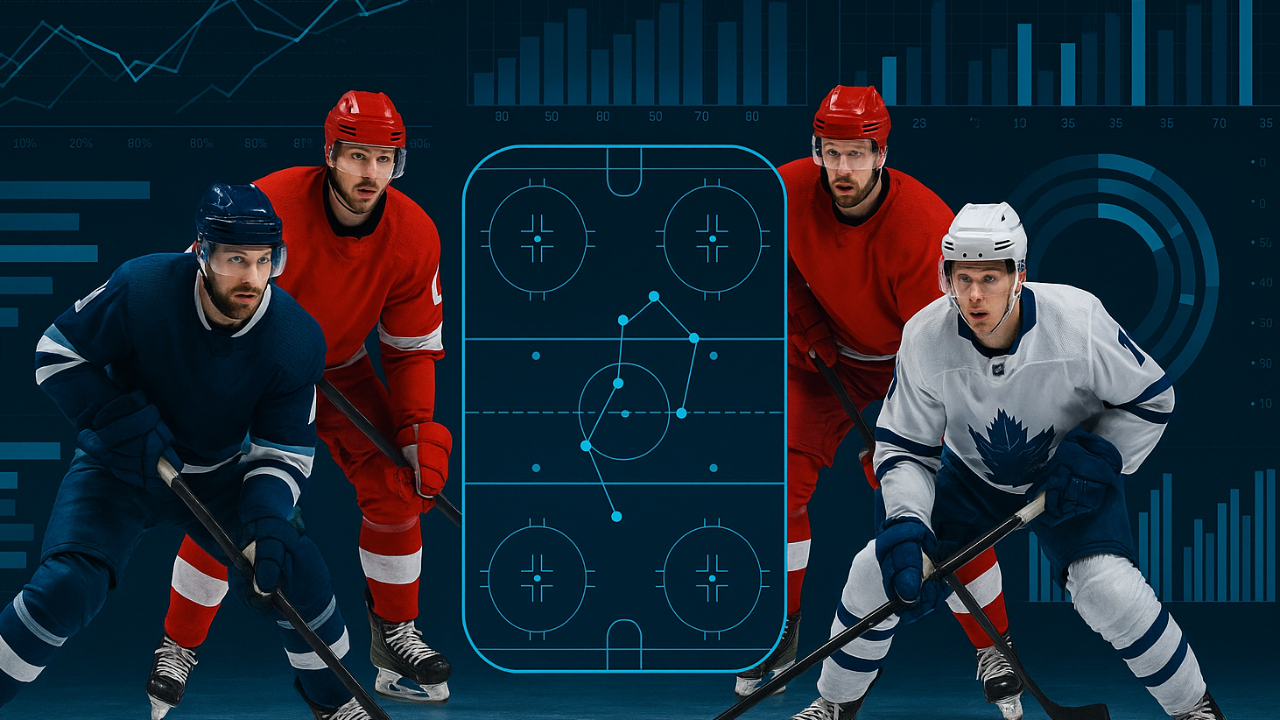
Our first article, “The Evolution of Analytics in Hockey – The Digital Twin,” explored how a digital twin can replicate players, tactics and even the ice surface itself, giving coaches a laboratory in which to analyze, predict and optimize performance with remarkable precision. This follow‑up explains how to build that twin and use it to craft winning game strategies for your next upcoming opponent.
How do we go about setting up the digital twin and how can it be used to determine and optimize game strategy for an upcoming opponent?
Let's start with the Data Collection process. We will focus on three types of data that the digital twin needs to simulate an upcoming game.
-
Athlete Data:Historical data on player performance against the upcoming team, historical game data of the upcoming team and even physiological sensor data (heart rate, speed, movement angles, fatigue patterns) if available. Sensor data is not needed to produce a digital twin but the more data points that are available for analysis, the more accurate the simulation will be with a higher confidence factor of the predictive capabilities of the model.
-
Environmental Data:Data about the playing environment (e.g., ice conditions).
-
Game Data:Player positions, game strategies used (e.g., breakouts, power play, penalty kill, neutral zone), shots per shift, goals per shift and other game-related data using manually tracked statistics, or even computer vision systems or tracking technologies.
Once all the data is collected, we can begin the Data Processing and Modeling step. Data modeling is the process of representing data objects and the relationships between them in a structured form that allows the digital twin to utilize the collected data to simulate a real-world event such as a hockey game.
-
Data Integration:Merge all data sources into a single, version‑controlled data lake (on premise or off premise) that maps every event to players, time and location.
-
Model Creation:Encode past player performance versus the upcoming opponent data using a simulation or analytics platform (dedicated sports‑twin engine, Power BI, Tableau). Use reduced‑order or machine learning surrogates so simulation runs finish in <1 s, fast enough for bench‑side queries.
Once the data stores and modelled, we can begin using the digital twin for Game Simulation and Analysis.
-
Scenario Simulation: Run simulations to test different game strategies. Stochastically vary 5–10 % of the model parameters on each simulation run to explore what‑if spaces: new breakout sequences, power‑play formations, unconventional line match‑ups.
-
Performance Analysis: Quantify the effect of each simulation on KPIs like shots‑per‑shift, expected goals, and zone‑exit success; convert these findings into targeted practice drills prior to the upcoming game with the specific opponent.,
-
Predictive Insights: Deploy the model to surface final in‑game recommendations—e.g., optimal face‑off alignment given score, zone, and fatigue state of players. The digital twin can predict potential game outcomes and risks at the play, system or overall game level. These predictive outcomes allow for proactive decision-making prior to the game and even on the bench during the game.
After using the digital twin and analyzing the output, we can begin the step of Continuous Improvement to improve the accuracy of the model's simulation capabilities and its ability to predict upcoming game outcomes by varying game strategies and player combinations.
-
Real-time Monitoring:Continuously monitor the performance of the digital twin and compare it to real-world data. Were the Digital Twin game strategy suggestions effective against the specific opponent? Which predicted events were over or under actual performance? Identify model issues and determine how the model can be modified to make a more accurate prediction in the future.
-
Model Refinement:Refine the digital twin based on new data and insights to ensure its accuracy and effectiveness. Feed discrepancies back into the twin, retraining weekly (or sooner) to keep pace with roster changes and tactical evolution. Model refinement would be done over the entire length of the season.
-
Optimization:Use the digital twin to identify training programs and practice strategies prior to an upcoming game that have the highest simulated ROI. The idea here is to use practice and training to maximize your team's performance based on game strategy suggestions prior to the actual game.
In the end, the Digital Twin will produce a comprehensive upcoming game strategy that can be used to place players in the best position to maximize shots per shift and goals per shift while considering various line combinations and overall playing time (e.g., Time On Ice). Remember, the twin is a decision‑support tool, not an autocrat; final strategy calls rest with the coaching staff’s experience and intangible reads. Used judiciously, the digital twin becomes the most powerful instrument in a modern coach’s toolkit.